What Are the Four Types of Analytics in 2023
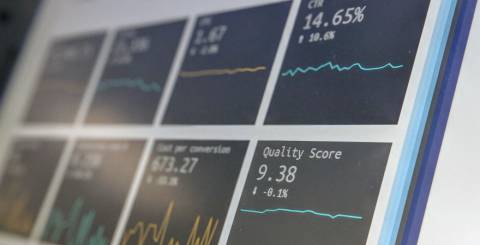
The landscape of data analytics is ever-evolving. Staying abreast of the latest trends and techniques is crucial for businesses seeking to extract valuable insights and make informed decisions. And, as we step into 2023, it's essential to understand the types of analytics and how they shape the data-driven world. They go beyond traditional descriptive analysis & embrace the power of advanced technologies to uncover patterns, predict outcomes, prescribe actions, and even automate decision-making.
With this blog, I'll explore each type and its unique capabilities while comprehensively understanding the analytics landscape and unlocking the potential for driving innovation and growth in the digital age. So, let's dive into the world of analytics and help you discover the transformative possibilities that await us.
The volume of data available to organizations today is staggering. When harnessed correctly, this data has the potential to drive decision-making, improve organizational performance and create an impact on strategy formation.
The following are the Advantages of Data Analytics
- Enhanced productivity and efficiency
- Improved financial performance
- Increased customer acquisition and retention
- Enhanced customer experience
- Better competitive advantage
Defining Analytics
It's the process by which data is manipulated to discover, interpret and communicate significant decision-making patterns. Data analytics can help reveal trends and patterns that might otherwise be lost in the mass of data and information.
Learning to analyze the data effectively is vital for organizations that want to harness the power of data and experience the benefits. Data analytics helps draw actionable insights, predictions and conclusions to make impactful decisions. There are more than just one type of analytics; each has a different story. Understanding analytics helps organizations select the right one that can benefit its business and drive business objectives.
Types of analytics
The analytics process is essential to apply and interpret the volumes of data that organizations collect. From customer behavior and revenue to business processes, analytics generates insights that can benefit businesses. Business analytics are of four types, descriptive, diagnostic, prescriptive and predictive. Understanding the difference and distinctions between each model can help learn how each analytics model can help businesses differently.
Descriptive analytics: Descriptive analytics examines, finds patterns, and spots trends from past data sets. This particular analytics method is used to answer "What happened?". This analysis method uses the documented past data available and generates information that businesses can quantify, evaluate and act following.
- Some examples of descriptive analytics include annual sales reporting, survey summaries, and annual reports.
- It can be used by an ecommerce business to analyze customer browsing and purchase history to make purchase recommendations.
- Financial organizations can use it to analyze and determine whether a customer is likely to pay card bills on time.
- Marketers can use it to analyze data to determine whether new customers will respond favorably or not to new product launches and campaigns.
While descriptive analytics is extremely useful to find patterns and trends, it cannot be used to go beyond past data to analyze historical data.
Implementing descriptive analytics: To implement an explanatory analytics program, repeatability and automation of processes are extremely necessary. Repeatability can be achieved when the data processes are standardized and applied routinely without effort, while automation would require zero manual intervention. The best way to achieve this is by adopting modern analytics tools that can help standardize and automate the back-end processes resulting in consistent user reports.
Diagnostic analytics: Diagnostic analytics helps explain and answer the question, "Why did this happen?". After finding out what happened or the reasons behind the performance, it is followed up with the diagnostics of why it happened and pinpoint the reasons behind the result. It is a handy tool for businesses to use to duplicate good outcomes and avoid bad ones. This analytical method's flaw or limitation is that it focuses on the past data.
Implementing diagnostic analytics: Modern analytics tools consist of various search-based AI capabilities. These features enable detailed insights. This is an important step, but tends to be skipped.
Predictive analytics: Predictive analytics aims to forecast likely outcomes and generate data-driven future outcome scenarios and tries to answer the question "what will happen in the future?". Using archival and documented data along with the present-day patterns makes it possible to make well-informed forecasts about the future business trend. It is common to use statistical modeling or machine learning with predictive analytics. The challenge with predictive analytics is that the insights it generates is limited to the data, which means that smaller or incomplete data sets may not generate accurate predictions. Some examples of predictive analytics can be -
- Calculate the risk associated with clients to determine the plans and rates offered.
- Using customer surveys and market research data to determine and identify the features that customers might prefer or find desirable when developing new products.
- Using predictive analytics to optimize patient care by assessing risk of patients developing specific health problems.
Implementing predictive analytics: Before using predictive analytics, some elements should be established, such as identifying a problem that needs to be solved. It is also necessary to define what the business is trying to achieve by using the predictions. Data preparation is an essential step which can be done by collecting the data, organizing it in a way that allows for data modeling, cleansing, reviewing and finally determining the objective.
Prescriptive analytics: This form considers all the possible factors to suggest actionable scenarios. Prescriptive analytics is all about what now or what is the next step. Various data sources are used, including machine learning, statistics, and data mining. Prescriptive analytics can also generate predictions related to future decisions and its ripple effects. Algorithms based on machine learning are used to go through large volumes of data to arrive at the optimal next step. Queries return a specific course of action. based on the logical if and else.
Implementing prescriptive analytics: Implementing prescriptive analytics starts after the first three analytical models have been established. This model is considered to be the combination of the first three analytical models, descriptive, diagnostic, and predictive. Moving step by step from each model brings the user to the prescriptive model.
In conclusion, using analytics is not new. Allied Market Research expects the self-service market for BI at USD 14.19 billion by 2026. Gartner predicts the growth of business-composed data and analytics to shift from IT to business. These four data analytics tools can give businesses the ability to make strategic, data-driven decisions. Data analytics managed services can help businesses turn data into actionable business intelligence and use the power of AI to work to create meaningful insights.
Similar Articles
Corporate transparency is essential in building stakeholder trust and credibility in today's evolving business environment. As businesses grow and adapt to changing regulations, ensuring adherence to rules and maintaining records has become increasingly intricate.
Among the solutions developed over the past few decades, Salesforce Financial Services Cloud (FSC) has emerged as the definitive choice for gaining flexibility, visibility, and long-lasting, inclusive growth in the financial sector.
Open source software (OSS) is distributed with its source code, which means it can be distributed, modified, and used freely with the original rights. Most users never see the source code, a critical part of the software.
It's one of the keystones, basic but key in the successful highly competitive modern business environment, where the connection with the customer is a must.
The speed of progress in the modern business landscape is quite relentless. For small-scale companies, this implies that keeping up with this progress is not simply gainful but fundamentally significant for their survival. And what does success in such an environment demand?
The finance sector needs to battle many difficulties in the modern and quick-moving digital landscape. Be it exploring the unpredictable snare of official guidelines or overseeing tremendous volumes of data - - financial establishments are feeling the pressure to succeed. This demanding environment, in turn, often leads to exhausted teams, costly manual errors, and inefficiencies that can be chalked up to repetitive tasks
The manufacturing industry, vital to the world economy, is at a pivotal intersection. I mean that, yet again, changes are afoot in the sector, this time driven by digital transformation as it represents a profound change in the very essence of how manufacturers operate, think, and drive innovation.
Technology helps make things easier and faster. Digitization is one of the aspects of technology that has changed how we live and work. It has brought many benefits for businesses, especially the travel industry. Customers can search online for the schemes offered and easily book trips, but payments need to be completed with ease.
In an article published by The Economist in 2017, while describing the astounding growth of titan companies like Google, Apple, Facebook, and Microsoft, it was mentioned how data had become “the oil of the digital era.”